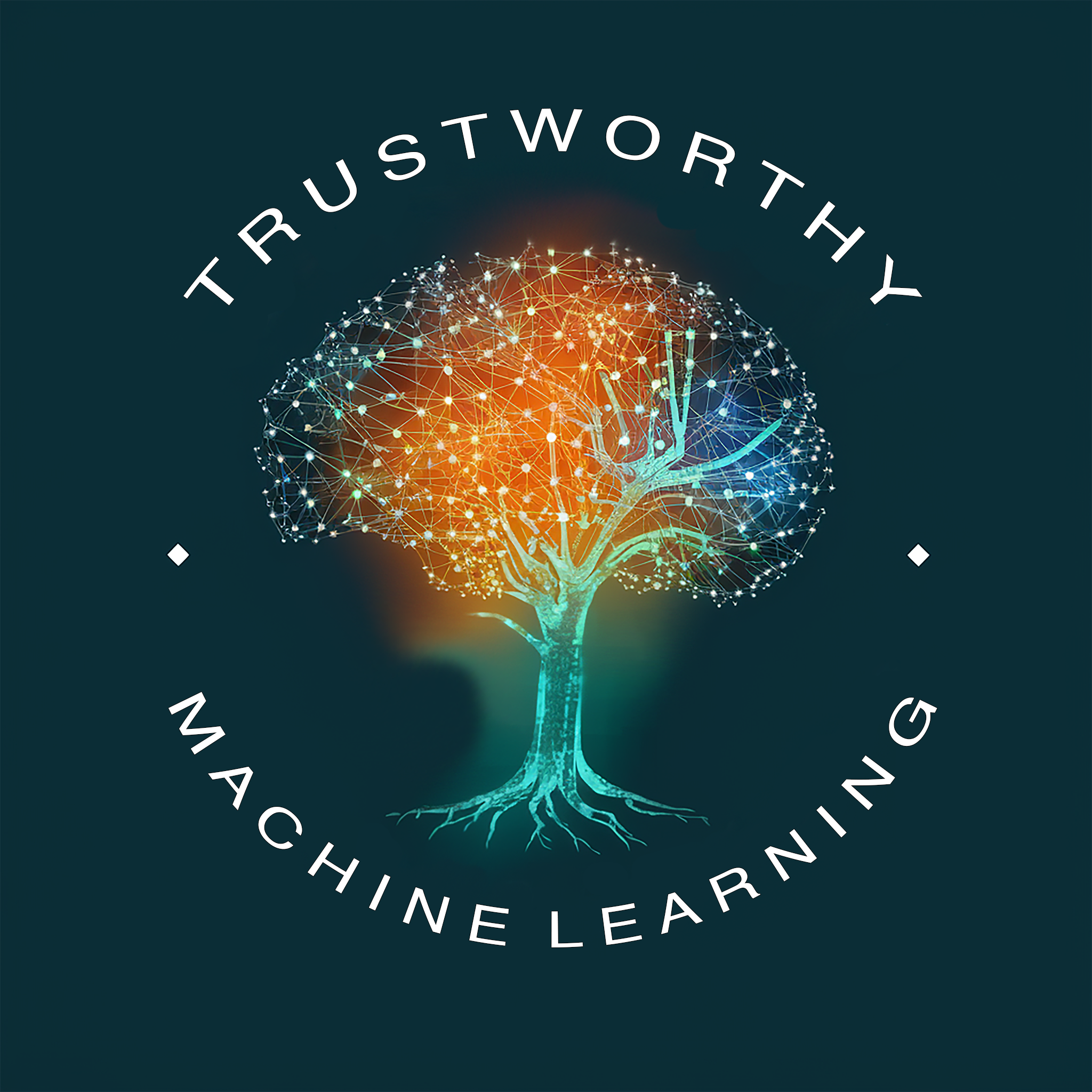
Machine learning has the potential for tremendous health innovations, but applying it in healthcare poses novel and interesting challenges. Data privacy is paramount, applications require high confidence in model quality, and practitioners demand explainable and comprehensible models. Ultimately, practitioners and patients alike must be able to trust these methods. In our research group on Trustworthy Machine Learning we tackle these challenges, investigating novel approaches to privacy-preserving federated learning, the theoretical foundations of deep learning, and collaborative training of explainable models.
Open Positions
- We offer Master and Bachelor theses for students within the UA Ruhr
If you are interested, please send an email to Michael Kamp.
Latest News:
-
Layer-Wise Linear Mode Connectivity
We presented our work on layer-wise linear mode connectivity at ICLR 2024 let by Linara Adilova, with Maksym Andriushchenko, Michael Kamp, Asja Fischer and Martin Jaggi. We know that linear mode connectivity doesn’t hold for two independently trained models. But what about *layer-wise* LMC? Well, it is very different! We investigate layer-wise averaging and discover…
-
Federated Daisy-Chaining
How can we learn high quality models when data is inherently distributed across sites and cannot be shared or pooled? In federated learning, the solution is to iteratively train models locally at each site and share these models with the server to be aggregated to a global model. As only models are shared, data usually…
-
Nothing but Regrets – Federated Causal Discovery
Discovering causal relationships enables us to build more reliable, robust, and ultimately trustworthy models. It requires large amounts of observational data, though. In healthcare, for most diseases the amount of available data is large, but this data is scattered over thousands of hospitals worldwide. Since this data in most cases mustn’t be pooled for privacy…